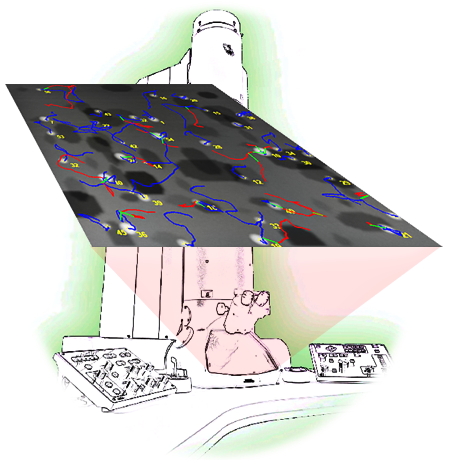
https://www.nature.com/articles/s41598-022-06308-2
This work, carried out with IRCELYON and the laboratory Hubert Curien, presents an approach combining deep learning and computer vision for the detection and the tracking of nano-objects in situ ETEM acquisitions. It allows the extraction of complex trajectories of small objects on a noisy and non-uniform background. Its application to the analysis of calcination of palladium nanoparticles on alumina support by ETEM electron microscopy, allowed to highlight coalescence and fusion phenomena very important for the catalytic properties.
After registration of the images of a sequence that drastically reduces the intrinsic spatial acquisition perturbation, a UNet is applied to detect nanoparticles. Then an original Multiple Object Tracking, optimized on the context of such nanoparticles trajectories, is applied to study their dynamic evolutions.
According to the few amount of annotated real data, UNet was pre-trained on synthesized data and fine-tuned on real data.
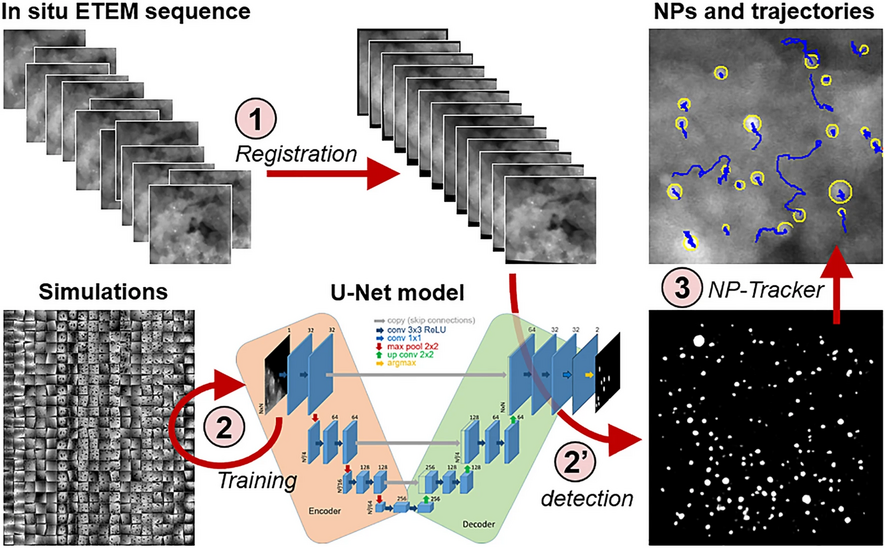